Multiple Comparisons
When the null hypothesis
The data can be arranged in a form of grouped data which is "grouped by" the column of categorical variable indicating factor levels.
Summary statistics must be calculated one level at a time.
Here we construct the confidence intervals simultaneously
for all pairwise differences
.
Then the point estimate of
and the standard error
are obtained respectively by
and
.
Various methods are proposed to find a critical point
so that we can obtain the confidence intervals
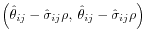


- Tukey's method.
Tukey introduced a studentized range distribution
's are independent standard normal and
is
-distribution with
degrees of freedom, independent of
's. Then we use
is given as the
-th percentile of the distribution for
.
- Scheffe's method.
As a special case of Scheffe's S Method,
we can obtain
- Bonferroni's method.
The Boole's inequality implies that we can choose
with
. Here
is the
-th percentile for student
-distribution with
degrees of freedom.
The significance tests for pairwise differences
are then performed in the following manners:
If the confidence interval for
does not contain zero, then we reject “
.”
The larger the critical point
is, the harder it is to reject
“
” (that is, the more conservative).
Remark on simultaneity. Whether we should conduct the analysis of variance (AOV) before multiple comparisons (MC) is a little sensitive issue, since it creates simultaneity of AOV and MC. However, because of the duality between the AOV and the Scheffe's S Method, a systematic approach popular among statistician requires the AOV in order to proceed with the MC. Also note that when we attempt different multiple comparison procedures (for example, Scheffe's and Tukey–Kramer's methods), naturally we do not discuss simultaneity of these procedures and understandably their conclusions may be inconsistent (for example, Scheffe's method may not detect any significance while Tukey–Kramer's method indicates significances for some pairs).
© TTU Mathematics